We have exciting new projects, not only for SA and MA students, but also other team
members
looking
for a fun and interesting project next to ETH obligations. If you are interested in a SA or MA
please
contact
one of our PhDs to discuss project ideas. For other projects, check out the list of current
projects
below
and
contact one of our TAs for more information.
If you are interested in previous projects, go to our Project Report site, where you can
download
multiple
reports of previous projects.
Bachelor / Semester / Master Projects
Please get in contact with us if you would like to do a project with us during HS23. The list of available projects will be updated soon. Long Term Tracking for Similarly Looking Objects in RoboCup Soccer SPL Games Distributed Optimization for Multi-Robot Systems Cooperation in RoboCup [closed] If you are interested in one of the projects above, please do not hesitate to reach out to the corresponding supervisors. For general questions about projects, please send an email to nomadz@list.ee.ethz.ch or submit your application here.
Freelancer Projects
We are always looking for freelancers to help us with our projects. If you are interested in working with us, please send an email to This is a glance of the projects we have to deal with as members of the team. Every year new challenges arise and our job is to find solution to them exploiting our capabilities while implementing the newest technological advancements to made our robots work better and better.
If you are interested in one of those or have other ideas, please contact us for more information. You can also join the team to immediately dive into the RoboCup experience.
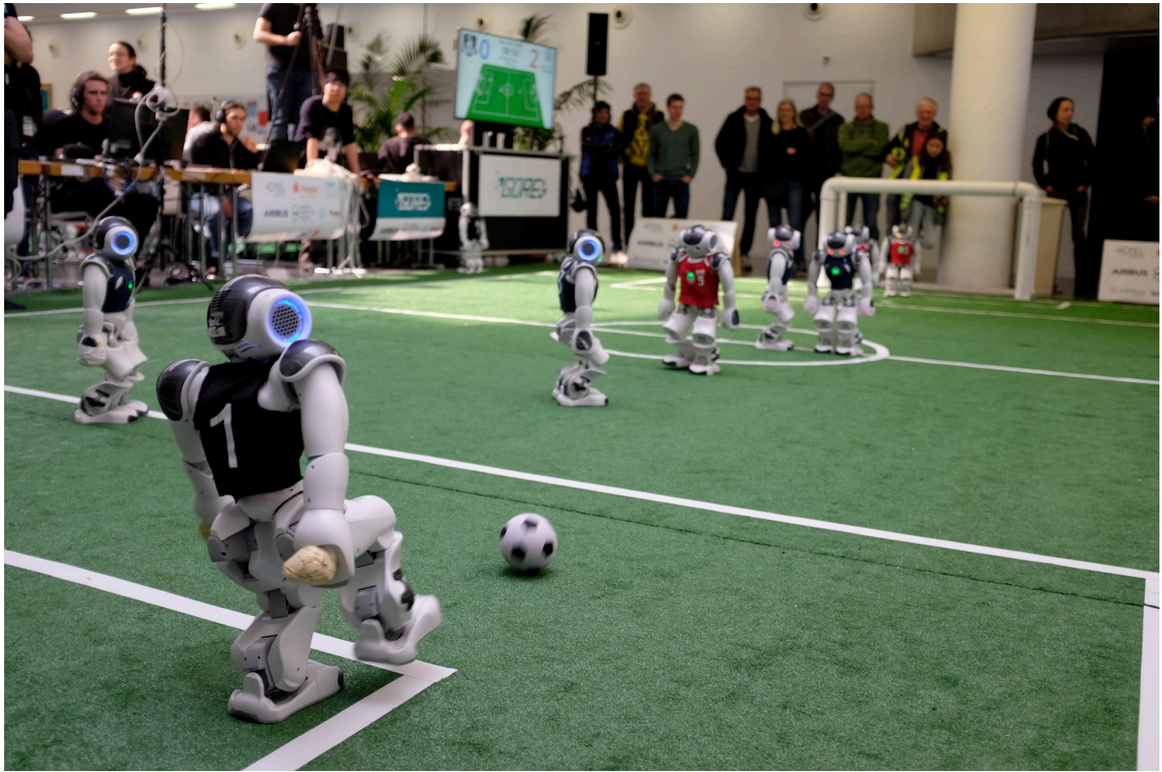
Control
Dynamic Kicking Skills for Humanoid Robots
Powerful yet accurate kicking is an essential skill for humanoid robots, especially in the context of robotic soccer competitions like RoboCup. Developing effective kicking skills for humanoid robots is a complex task that involves a combination of mechanical design, advanced control algorithms, and sensor integration.
This project aims to develop a robust control system for a NAO robot to successfully kick a rolling ball into a designated goal area. The system will leverage Model Predictive Control (MPC) for trajectory planning and Moving Horizon Estimation (MHE) for state estimation. MPC allows the robot to predict and optimize its movements to achieve the desired kicking motion, while MHE helps in accurately estimating the robot's and ball’s current state based on sensor data. Both components are crucial for making real-time adjustments to the kicking motion.
Decentralized Global State Estimation for Humanoid Robot Soccer Teams
In this project, we aim to develop a decentralized global state estimation and predictive decision-making model for a team of humanoid robots playing soccer. Each robot in the team will locally construct and update a global state representation, including robots and ball location, by fusing multiple streams of sensory and communication data.
Currently, state estimation is mainly done through robot odometry, providing position and orientation from proprioceptive information. The estimate is then adjusted using a vision-based localization from landmarks detection. Additional information to be used are the teammates and opponents detection, and an internal communication between players, which helps sharing important updates. The resulting world model should then be used to optimize real-time decision-making during the game, enhancing team coordination and individual robot performance. Ideally, a combination of Model Predictive Control (MPC) and Moving Horizon Estimation (MHE) methods should be employed.
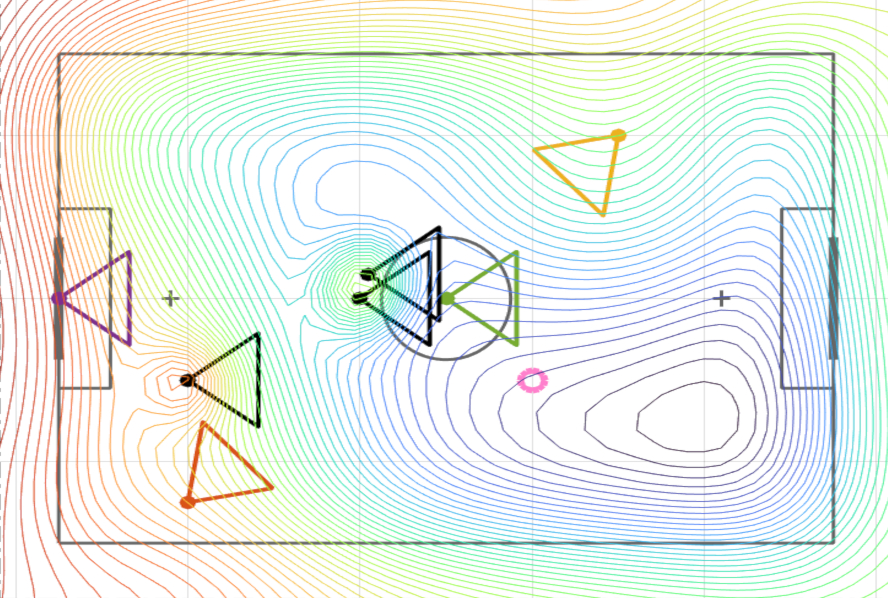
Behavior & Decision-Making
Behavior
The current behavior is relatively old and contains many issues that have yet to be solved, but until now there were many other more pressing issues that needed to be solved.
Now with the SelfLocator, Ball Detection, Robot Detection, and most basic motion working reasonably well, the implementation of more advanced behaviors starts to make sense.
The final goal of this project is to enable the robot to pass the ball to each other and incorporate this skill into offensive and defensive gameplay. Theoretically, this is fairly simple but in practice, it is very hard to execute since most kicks are inaccurate, opponents are usually all over the place when trying to execute this maneuver, and most importantly all estimated states and information you have are not 100% correct.
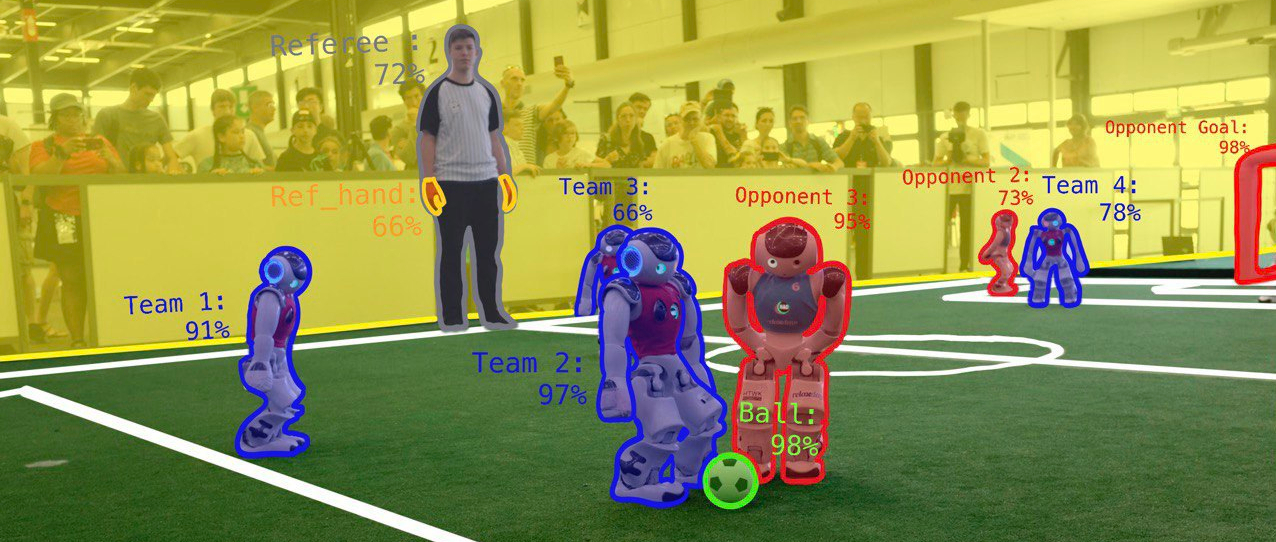
Computer Vision
Team Detection
Detecting robots is one of the main vision tasks in a robot soccer perception stack. Currently, our framework uses a single-stage object detector based on the SSD meta-architecture to detect robots but unfortunately at the moment we are not able to determine the team to which the robot belongs i.e. our own team or the opponent team. While this is already useful for obstacle avoidance, it is not good enough to implement collaborative behaviors.
In this project we are going to design and implement an approach to determine the team of a detected player based on its jersey color. We are going to start with classic computer vision techniques using color based heuristics and eventually move to a more robust learning-based approach.
Sim2Real Translation
Deep learning models have become the standard for perception in SPL, especially for tasks such as object detection and scene understanding. However, collecting high-quality data with the hardware of the NAO and annotating it is a tedious and time consuming task.
One possible workaround is to use synthetic data. State-of-the-art game engines such as Unreal are capable of rendering highly photorealistic scenes, which can then be used to generate synthetic dataset. However, training only on synthetic data might result in poor generalization.
In recent years, it has been shown that deep learning can be used to transfer the properties of a dataset to another one with the same labels but different domains e.g. real and synthetic robot soccer scenes. In particular, models belong to the CycleGAN family have shown very promising results.
The goal of this project is to set up a pipeline to automatically generate annotated synthetic training data for computer vision models from robot soccer scenes rendered e.g. using Unreal Engine and make it more “realistic” using a style transfer model training on publicly available datasets.